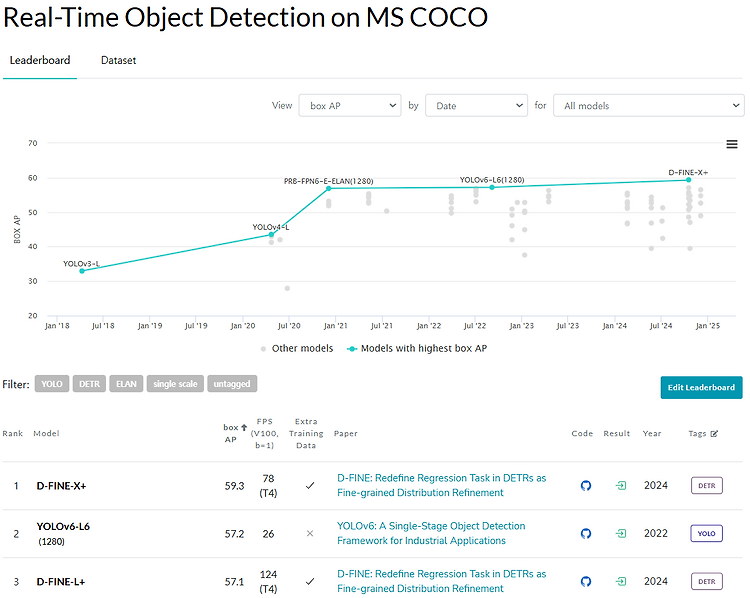
D-FINE: Redefine Regression Task in DETRs as Fine-grained Distribution Refinement
·
Study/computer vision
D-FINE: Redefine Regression Task in DETRs as Fine-grained Distribution RefinementPeng, Yansong, et al. "D-FINE: Redefine Regression Task in DETRs as Fine-grained Distribution Refinement." arXiv preprint arXiv:2410.13842 (2024).RT Detection SOTA D-fine에 대해 리뷰 진행.AP와 Latency 사이에서 둘 다 굉장히 우수한 DETR 기반의 RT Detector이다.아마도 이 정도의 FPS와 box AP의 성능이라면,End-to-End 방식이라는 점과 결합하여 Yolo를 밀어낼만한 충분한 detector라고 생각한..